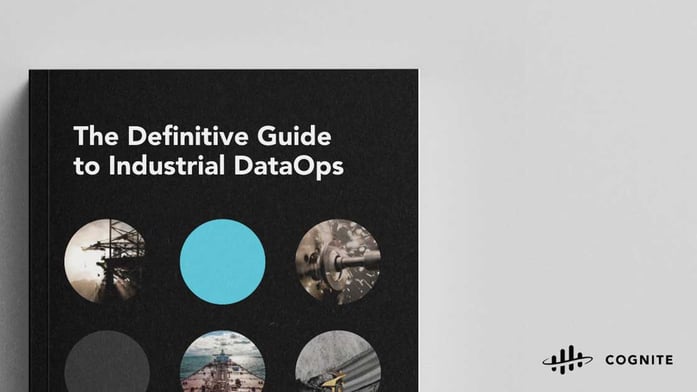
2020 forced certain industrial sectors, once seemingly invincible, to grapple with their mortality. The economy was shattered. Oil prices fell below zero for the first time in history. Millions of jobs were lost.
Worst-case scenarios were no longer just hypotheticals—they would be distinct possibilities without massive efforts to transform, address vulnerabilities, build resilience, and digitalize.
Suddenly, once-analog traditional industries like oil and gas, power and utilities, and manufacturing began adopting digital tools at a faster pace, from remote work to robotics.
Many of these initiatives quickly ran into a roadblock.
For too many industrial companies, data is still inaccessible and disconnected. It takes more time to find the data than to build solutions that create value. Compare that to the consumer world, where data is instantly accessible anywhere, anytime, and applications use machine learning to learn from our behavior and make recommendations.
To solve this fundamental data problem in industry, we need new ways of working with data. One absolutely essential tool is the new, rapidly growing discipline of data operations (DataOps).
What is DataOps?
DataOps in 2020 is as new and unknown as DevOps was in the 2010s, business intelligence in the 1990s, and human resources in the 1890s.
DataOps can be defined as a new discipline which typically comprises a team of data experts (think: data scientists, analysts, architects, and the like) who exist to “provide the tools, process and organizational structures to support the data-focused enterprise.”
DataOps engineers and teams are tasked with bringing data to data customers throughout the organization, internal and external, and making their data do more for the company.
The term DataOps dates back to the 2010s. In a 2014 blog post, Information Week contributing editor Lenny Liebmann looked at the emergence of big data and saw a need for a set of best practices to ensure that data science happens efficiently and reliably.
Liebmann called this discipline “DataOps,” or “the set of best practices that improve coordination between data science and operations.”
Seven years later, DataOps is a “top trend,” according to VentureBeat, and the race is on to sharpen the definition and claim a piece of the market:
-
According to Gartner, “DataOps is a collaborative data management practice focused on improving the communication, integration, and automation of data flows between data managers and data consumers across an organization.”
- A team of Forrester analysts, including Michele Goetz, vice president and principal analyst, defines DataOps as “the ability to enable solutions, develop data products, and activate data for business value across all technology tiers from infrastructure to experience."
Two strong common threads across these definitions are the importance of collaboration and the focus on integrating data across different parts of an organization.
DataOps vs. DevOps
DataOps, DevOps, MLOps—it can be difficult to keep track of the latest buzzwords out of the world of IT. Here’s a clarification:
DataOps is not DevOps. DevOps has been around for years. Despite some superficial commonalities, DevOps and DataOps are fundamentally different. Both are methodologies used to enhance operational practices, but that’s where the similarity ends.
The focus for DataOps is the delivery of business-ready, trusted, actionable, high-quality data, available to all “smart engineers” or “data consumers.” One goal is automation efforts, centered on data governance and integration. Another goal is alignment between IT system support, operations, and the business.
The focus for DevOps, in comparison, is software and application development. Automation efforts center on the development cycle, software delivery processes, and waste elimination. The alignment of developers, operations, and the business is a major aim of DevOps.
What is Industrial DataOps?
A more specific version of the new discipline, Industrial DataOps is about breaking down silos and optimizing the broad availability and usability of industrial data generated in asset-heavy industries such as oil and gas, power and utilities, and manufacturing.
Here are three key truths about Industrial DataOps:
- Industrial DataOps depends on collaboration with domain experts.
Individuals and interactions (far more than processes and tools) are essential to make data valuable and useful for data consumers across an organization—in other words, the domain experts in different fields and departments.
It’s important to remember that DataOps is a practice, a way of engaging and collaborating across the organization to both share and reap greater value from the data.
- Data is only as valuable as the analytics behind it and the scale of people who use it.
The convergence of data and analytics has made Industrial DataOps an operational necessity. To be used broadly, data requires context. Automating the data process and creating one central, contextualized source of truth is the only way to make sure that live data triumphs over static documentation and reports in the decision-making process. - Extracting the value of the data requires an agile approach.
Industrial DataOps isn’t about documenting, reporting, or extensive up-front design. It’s a far more agile process in which experimentation, iteration, and feedback are essential.
Creating business value isn’t a one-way transaction between data scientist and department. It’s a joint effort that requires both sides to participate, share, and develop solutions that hold transformative potential. The data is alive, and so are the means of working with it.
What are the benefits of Industrial DataOps?
Industrial DataOps is already becoming a driving force in industrial transformations, helping accelerate digital maturity, enabling data teams to deliver more digital products, and realizing more operational value at scale.
In a 2020 survey of global companies, McKinsey found organizations that embedded DataOps could see the volume of new features increase by 50% because data automation enables quicker development iterations.
Specific benefits include:
-
Efficient data management: DataOps maximizes the productive time of data workers with automated data provisioning, management tools, and analytic workspaces to work with and use data safely and independently within specified governance boundaries.
- Improved data accessibility: DataOps technology uses AI to enable rapid ingestion and contextualization of large amounts of data.
- Rapid use case development: DataOps aims to shorten the time to value of data by making proofs of concept (PoCs) quicker and cheaper to design, offering tools to operationalize and scale them.
- Enterprise data governance: DataOps enables companies to set and enforce the basic principles for managing data, providing consistency and ROI in technology, processes, and organizational structures, with better operations data quality, integration and accessibility, and stewardship.
Are you ready for Industrial DataOps?
Any organization that wants to adopt and enjoy the benefits of Industrial DataOps needs to consider the state of its digital maturity.
Achieving high digital maturity is important to deliver greater return on investment, operational efficiency, and commercial success. Industrial DataOps provides a way to digitally mature and realize these goals.
Take our digital maturity calculator now to find out how prepared your organization is to improve operational performance with data and analytics at scale.
Answer these 20 quick questions to find out how digitally mature your organization is, benchmark your score against industry peers, and receive recommended actions.