The manufacturing industry doesn’t need more data. It needs more context.
It’s easy to be disillusioned about digitalization these days. Over the past five years, the manufacturing industry has seen mostly disappointing results from machine learning and data science initiatives. These much-hyped initiatives once promised predictive maintenance and improved performance. Instead we’ve found them to be inefficient, costly, and difficult to scale.
Why? It’s not because manufacturers aren’t collecting enough data. Data lakes have become commonplace in the industry, yet even with all the data they need in one place, data scientists are still spending about 80% of their time on collecting and cleaning data — not on running advanced analyses and refining algorithms that generate value.
.png?width=717&name=Main%20point%20long%20(1).png)
Fixing this issue requires a new approach to data operations (DataOps), a collaborative data management practice focused on improving the communication, integration, and automation of data flows between data managers and consumers across an organization. Simply put, DataOps means getting the right data to the right user with the right context for the right problem at the right time.
Read also: The top digitalization trends and opportunities changing the manufacturing industry
That work starts with investing in data contextualization. By combining insights from different data sources and types, manufacturers can empower their engineers with real-time information, democratize knowledge previously only available to experts, and rapidly develop and scale new and existing applications.
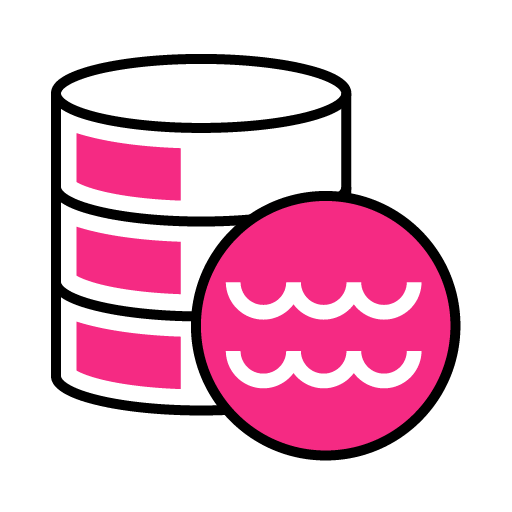
The Problem with Data Lakes
Whatever happened to unlocking infinite data-driven potential by liberating data from siloed source systems and integrating it all into one single repository? Let’s examine the anatomy of the paradox.
First, data lakes only store data in an untransformed raw form.
While raw data is theoretically available across realms of immediate, potential, and not-yet-identified interest, active metadata management is often an afterthought. It winds up as the technology project’s flagship KPI but lacks enthusiasm or investment from the stakeholders along the way.
Raw data — absent of well-documented and well-communicated contextual meaning — is like a set of coordinates in the absence of a mapping service.
Those lucky few who intuitively understand the coordinates without a map may benefit. For all the rest, it’s the map that provides the meaning. Without a map, coordinates alone are useless to the majority.
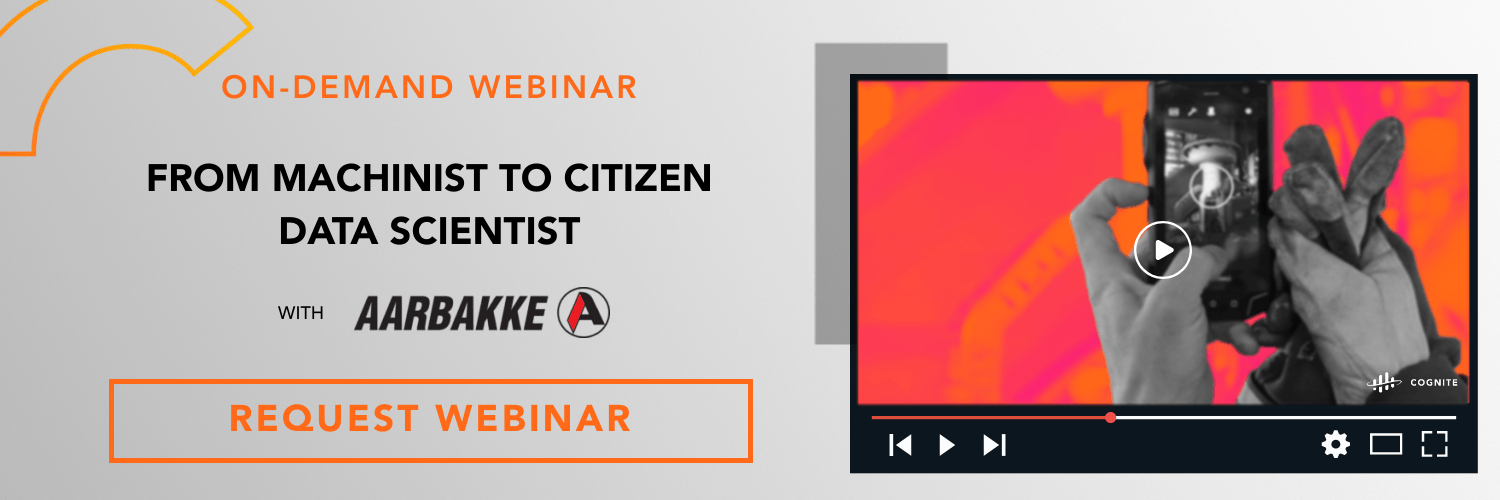
Second, data lakes lack contextualization.
While some applications benefit from raw data, most applications — especially low-code application development — require data that has undergone some additional layer of contextual processing. This includes aggregated data, enriched data, and synthetic data resulting from machine learning processes.
Here is where the value of data contextualization becomes most pronounced. Aggregated, enriched, and synthetic data delivered as an active data catalog is far more useful to application developers. Strong API and SDK support, designed for use by external data customers, further amplifies the value of this processed data. It’s also something raw data in a large, unified container fails to address.
Data lakes that only store data in an untransformed, raw form offer little relative value. These vast amounts of expensively extracted and stored data are rendered unusable to anyone outside the data lake project team itself. (And too often remain somewhat useless to that team, as well.)
Read: Reaching a Critical MaaS
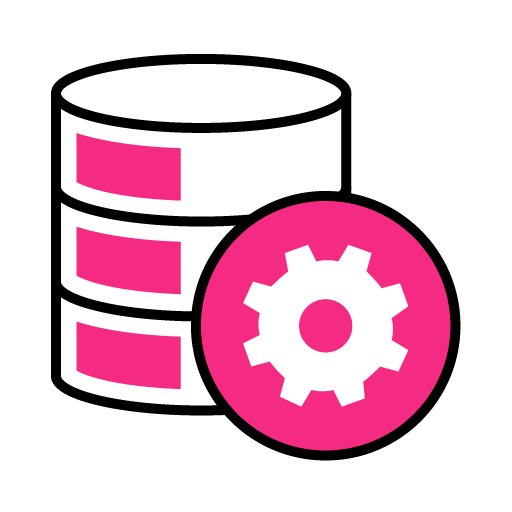
Adding Contextualization to Existing Data Lakes
Data contextualization goes beyond conventional data catalog features (see table below) by providing relationship mining services using a combination of machine learning, rules-based decision-making, and subject--matter expert empowerment.
Many mid-sized manufacturers, operating mostly with IT data, may benefit from a simpler data catalog solution. However, large industrial asset operators dealing with the synthesis of OT and IT data — not least the ongoing proliferation of IoT data together with very complicated brownfield data realities — call for an enterprise-grade data contextualization solution.
Contextualized data generates immediate business value and significant time-savings in many industrial performance optimization applications, as well as across advanced analytics workstreams.
By liberating data from their silos, defining the relationships between them, and making it all available in the cloud, manufacturers create a foundation on top of which they can build both advanced and low-code digital tools that make insights available across the organization, enabling remote monitoring and diagnostics. This lets engineers focus on solving operational problems, improving existing products and services, and developing new solutions.
The distance between a data lake and a digital twin may not be that far after all. Contextualization, offered as a service for data already aggregated in one place, offers an instant upgrade.
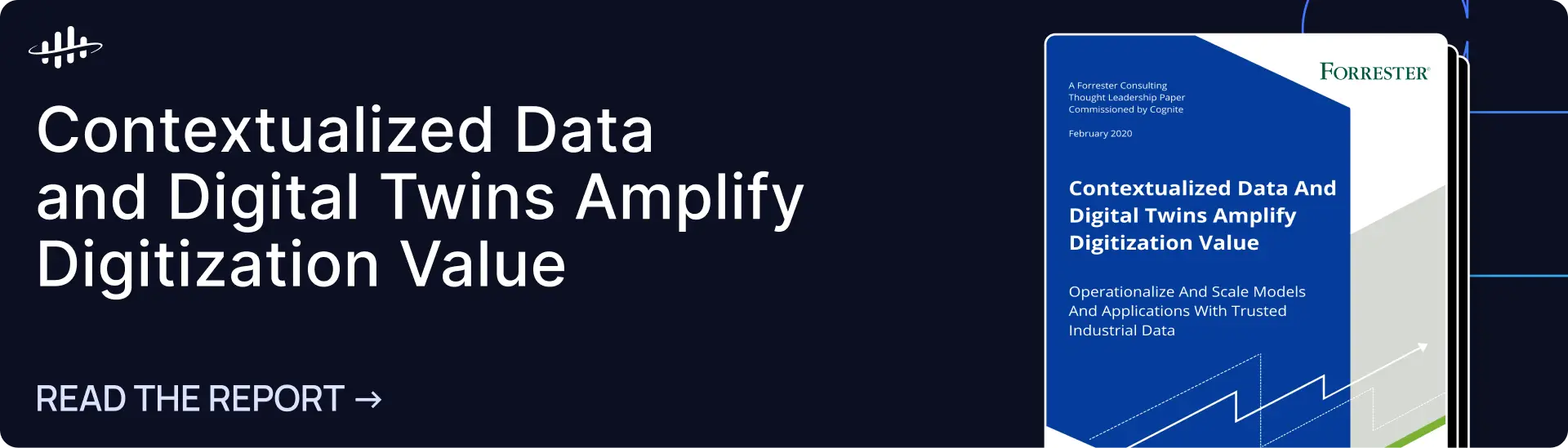