Controlled water flow is the obvious center of all hydropower operations. This is where stored, potential energy transforms into valuable, sustainable power — a source which accounts for more than 1300 GW of electricity produced worldwide.
Hydropower operations have been evolving through technology, process, and approach in order to control, optimize, and take advantage of this water flow. With the same end objective in mind — improved operational efficiency and adaptability to compounding energy industry pressures — operators can take a similar approach to data flow and transformation.
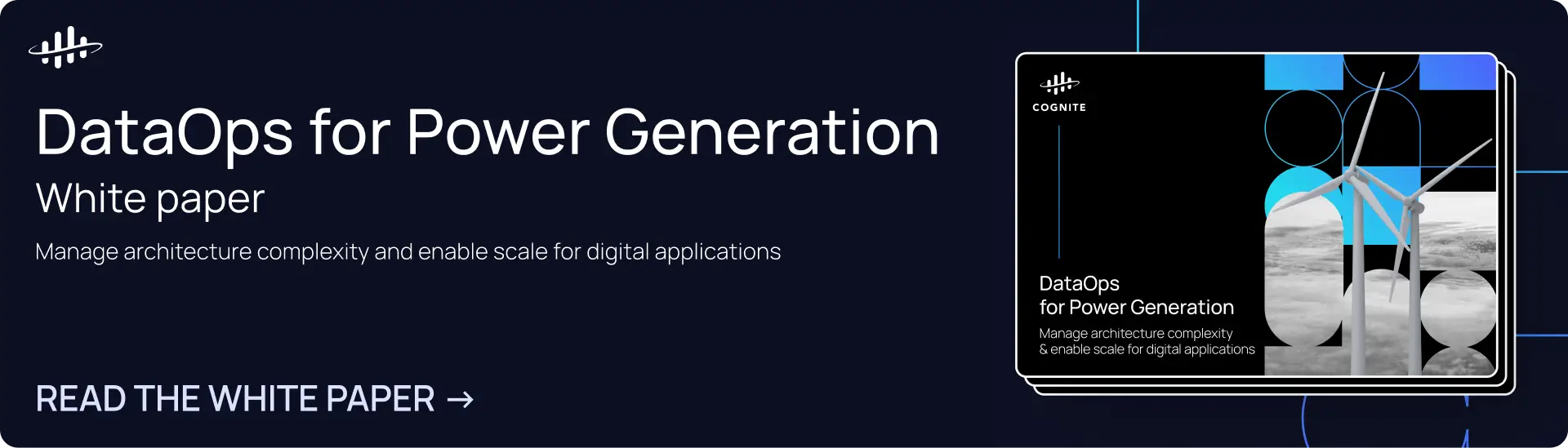
In this post, you’ll learn three key points about digitalizing and maximizing hydropower operations:
- The root causes of stagnant data flow and the valuable implications for digital operations once data moves freely about the organization
- Strategies and tactics to get data flowing and operationalized
- How to minimize risk and avoid the pitfalls that risk derailing the digital transformation journey
Today, it is undeniable that industrial data represents a tremendous amount of “potential” value, literally being stored in data “reservoirs” (to continue the analogy), and needing to be dispensed and consumed at will across modern organizations in order to deliver value. But decades into digital transformation, Gartner reports that up to 97% of data still goes unused in organizations. For many operators, what is meant to be a data flow is in reality just a trickle.
With daily data creation far outpacing data consumption via AI, advanced analysis, or other digital applications, it is hard to imagine how operators can start to control the data flow from these reservoirs without expanding their usage of technology and automation, both key tenets of modern data operations (DataOps) practices.
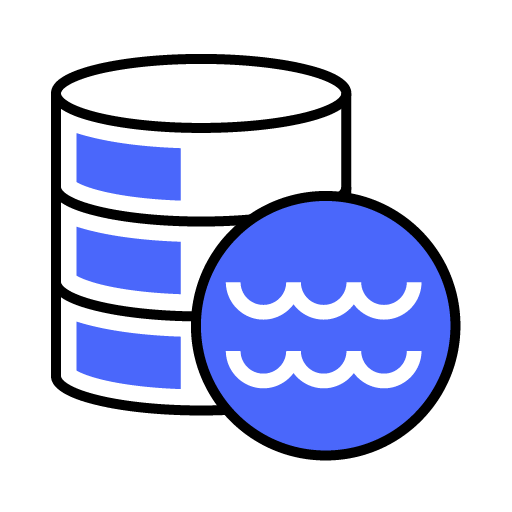
Avoiding the Data Swamps and Whirlpools
Swamps form when water stagnates and carries no movement or flow, leading to unpleasant, harmful environments.

Take for instance the process of building a predictive model to predict hydro generator failures — if it takes 3-6 months just to access, clean, and operationalize the data for modeling, will the model even be relevant in the deployed season and later in that asset’s life cycle?

Data pools into swamps when it can’t be easily accessed on demand at the right time. In some cases, this is due to physical access where the data is either unknown to the user or restricted in a siloed database governed by a different department. As IT and OT converge, for example, data from these historically different domains can and should be used together to make better informed decisions — but is your organization set up to facilitate this exchange?
In other cases, restricted access is a function of context — in that the end user must not only be an expert in the business problem and methods of analysis, but also an expert in the data process, source, structure, and hierarchy. Data without automatic context can further stymie the flow and progress of a project, contributing to high-overhead “whirlpools” where more resources are consumed in preparing and contextualizing the data than to solve the business problem itself.
Stage 1: From Data Trickle to Data Stream
Opening the data “spillway gate” in a controlled manner doesn’t have to be complicated, but it does depend on having the right infrastructure in place.
When preprocessed data can be accessed with less effort and overhead, it can be put to use quickly and repeatedly in dashboards and reports, delivering a new level of operational visibility that drives improved decision-making. And with more transparent data collection, curation, and distribution processes, the quality of data improves so that the data model can be depended on as a single source of truth.
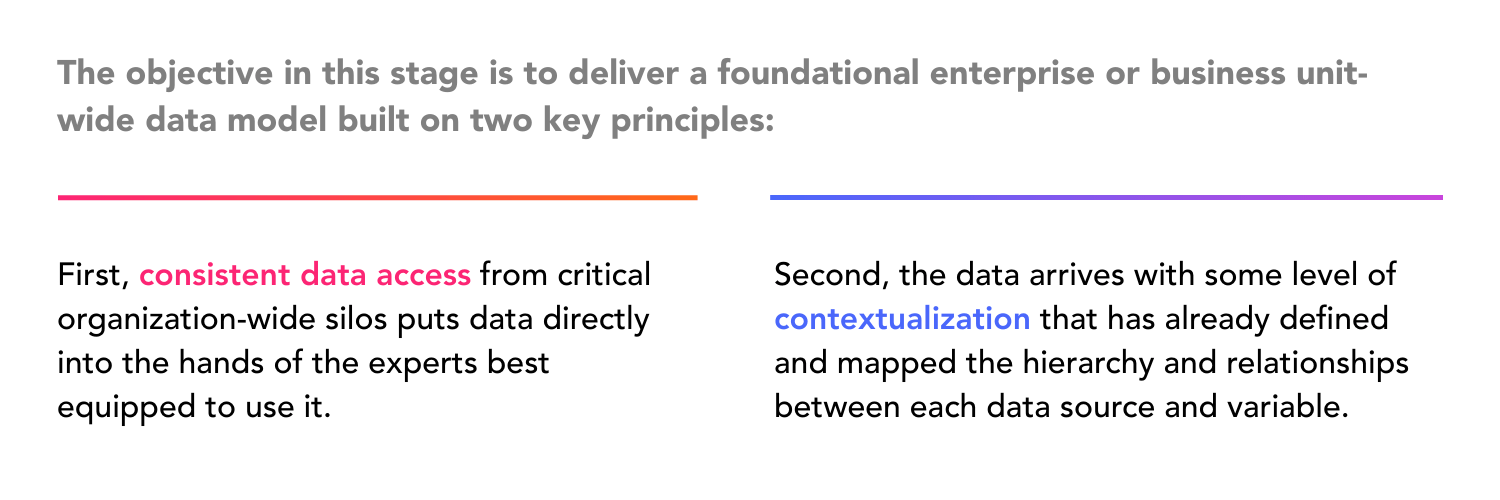
For a hydropower operator, this enabling architecture starts with a robust library of off-the-shelf connectors and extractors to securely access SCADA systems, control system data, event logs, work order management systems, and more, and the user experience to see these data streams in a single location. Additionally, this architecture must include checks for data quality and the ability to transform, contextualize, and enhance raw data into meaningful information that can be represented in dashboard form via APIs and SDKs.
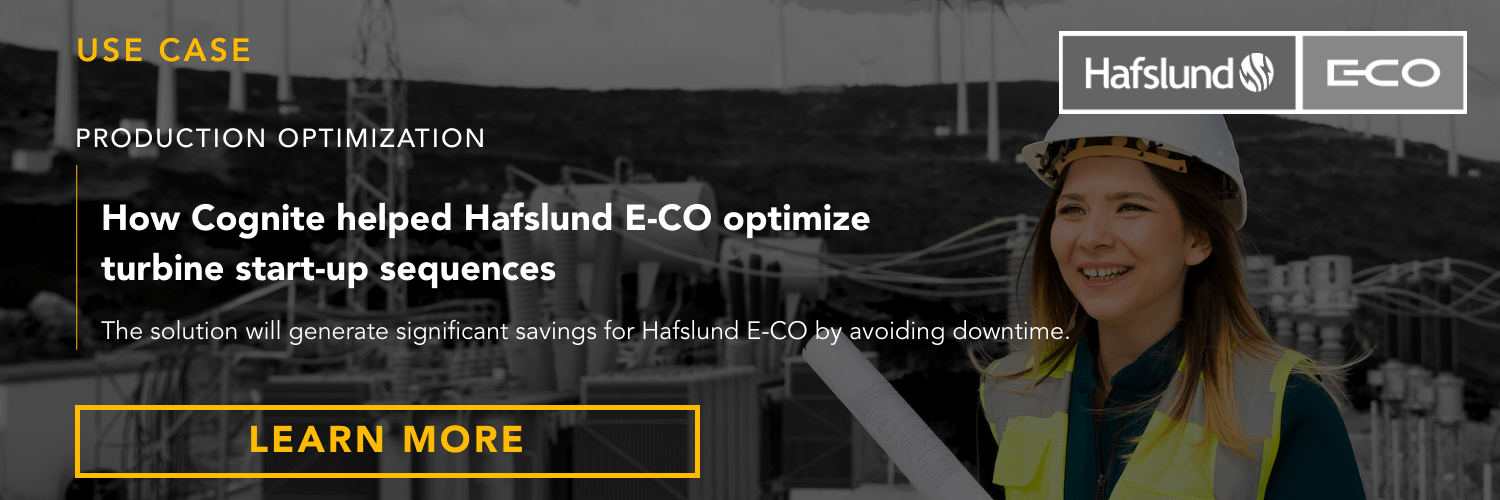
By solving for the fundamental issues with data access, quality, and contextualization, hydropower operators can realize short-term success, measured by day-to-day decision-making quality, accuracy, and timeliness, and longer-term success, measured by advancement and optimizations in workflow and automation.
Stage 2: From Data Stream to Data Pipeline
Now that data is flowing in a powerful, yet unstructured stream, hydropower operators can further transform the flow into systemic value capture by developing and scaling digital applications that radically evolve entire workflows and business models. This involves creating and operationalizing the predictive models that bring clarity to and automate decision-making processes.
Listen to how a Norwegian hydropower operator, Glitre Energi, is amping up its own technological transformation by building deep tech competences and the power of prediction in this episode of Cognite Convos:
When data is contextualized and served to data scientists in a more meaningful way, data scientists spend less time sourcing and cleaning, and more time on modeling. A robust data model also facilitates the inclusion of nontraditional data that can make a predictive model much more accurate and insightful.
For example, time series data has become an industry standard for delivering predictive asset failure models. But what if you could also include additional data such as demand patterns, weather data, and overall maintenance history — all from various siloed sources? This would result in a much more accurate and explainable model because it is based on all available data rather than a myopic fraction that the data scientists and subject-matter experts are already familiar with.
In many cases, operators look to run with advanced analytics and machine learning before they can walk due to the promised appeal of such technologies. But because these applications consume so much data, few are truly ready to operationalize at scale due to the nuance and complexity involved.
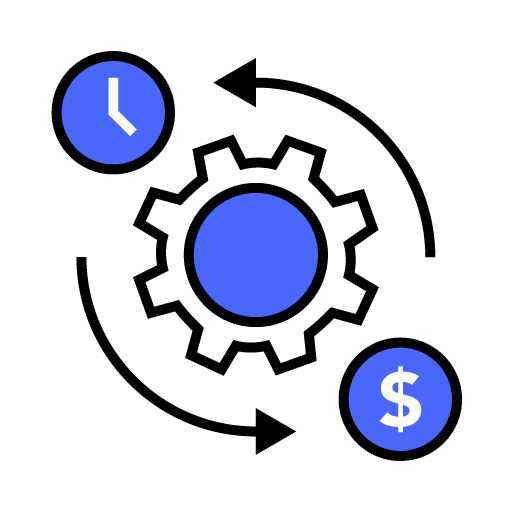
Avoid Freezing the Flow
As digital maturity in the hydropower industry accelerates, new examples are emerging of how data flow can be negatively impacted by organizational change. For hydropower, this commonly results from the competitive nature of digital solution providers who create vendor lock-in by ingesting — and then claiming ownership of — the data related to their application. While vendors can solve individual problems, few (if any) are equipped to solve the holistic problem set for each of their customers.
This creation of additional silos and digital complexity carries the very real risk of driving up your human costs of data management and reducing overall ROI, effectively “freezing over” your data stream and reducing your data flow rate. In competitive energy markets, operators must continue to push the digital envelope in order to harness the competitive advantage of their data flow.
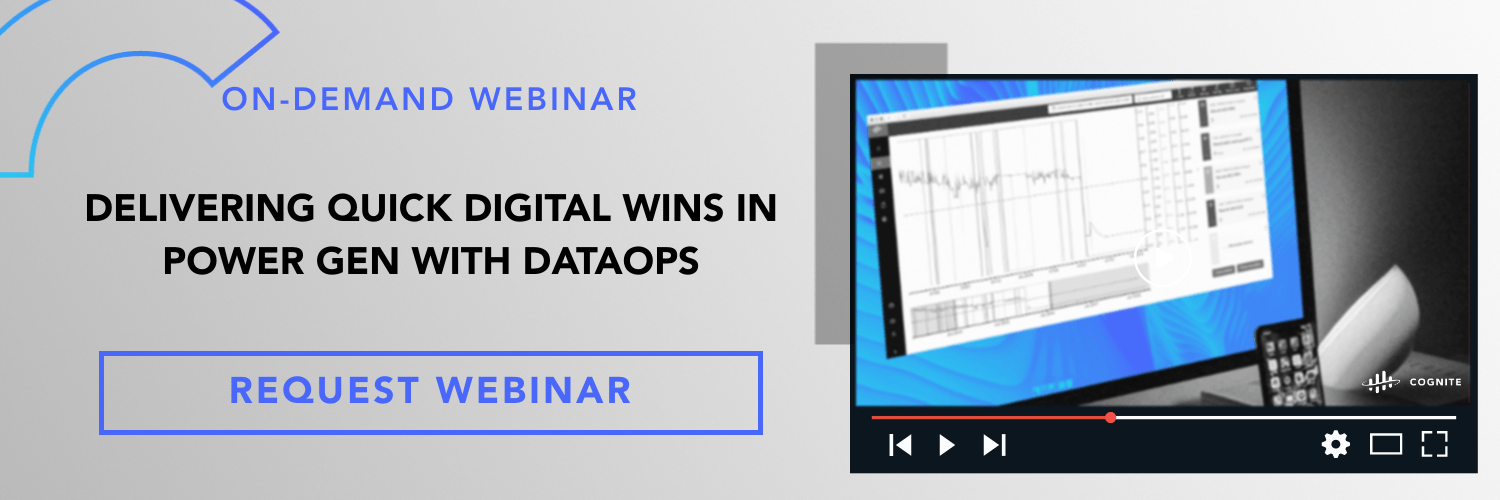
Much like controlling water flow rate, maximizing hydropower operations with an effective data flow and control comes down to infrastructure, technique, and expertise. Cognite’s DataOps solution offers a secure means for hydropower operators to democratize these elements so that more users can leverage data for higher impact at lower marginal (and human) costs. This paves the way for the rapid adoption of innovative digital applications around remote work, predictive maintenance, and autonomous operations (among many others). Without a strong data foundation, hydropower operators will struggle to adapt to the unprecedented change in today’s power market.
Don’t get stuck in the data swamp. Let your data flow freely across your organization.