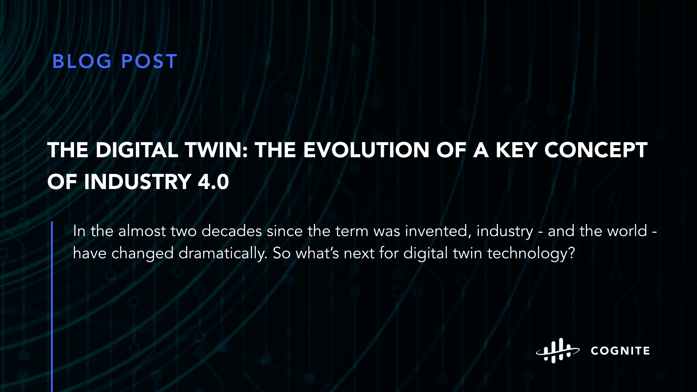
The digital twin is the foundation for industrial digitalization efforts, delivering real-time insights, accurate forecasting, and intelligent decision-making. In the almost two decades since the term was invented, industry - and the world - have changed dramatically.
So what’s next for digital twin technology? Johan Krebbers, IT Strategist at Cognite, summarized his perspective on the evolution of the digital twin concept during a panel at Ignite Talks, 2021’s big industrial digitalization conference. Read below an extended expert interview between Johan and Petteri Vainikka, our Vice President of Product Marketing, on the future of digital twins.
Hello, Johan! Thank you, for taking part in our panel at Ignite Talks and especially for taking the time to do a deep-dive interview to expand on your contributions to the panel! Let’s start with a lightning round question. All I need is a simple yes or no. You’ll get to elaborate in a second. Should we sunset talking about digital twins and simply call it industrial application development?
No.
Can you elaborate on why you answered yes or no?
To me, the digital twin is foremost about the data integration layer. This can play a critical role in all application development, especially focusing on industrial production optimization and smart maintenance. However, the term digital twin deserves to be retained in order to highlight the unique role that data integration - or data contextualization, as it is often referred to within industrial data management — plays.
The simplified tech stack needed for application development consists of a data layer, an analytics layer, and a low-code layer for visual app development. Thinking beyond proofs of concept, this translates into a DataOps layer, a ModelOps layer — including simulation hybrids — and a DevOps layer. On a scale of 1 to 5, where would you put the importance and maturity of each layer today?
Before answering, I again want to highlight the role of OT/IT/ET/visual data integration in the first layer, which you categorize as the data layer, or the DataOps layer. To me, this is the most important layer to get right, in order to achieve success with digital twins. There are very few companies today who can bring together, in a meaningful and efficient manner, all these different datas needed for digital twins. Cognite is at the very front of the shortlist.
Figure 1: Industrial data operations makes industry 4.0 work. Cognite Data Fusion® is the Industrial Data Operations platform for digital transformation.
When it comes to the other two layers in the tech stack - the analytics layer (the ModelOps layer) and the low-code app development layers (the DevOps layers) - they are both much more mature and crowded, with plenty of strong solutions to choose from, based on needs and priorities.
What is very important is that, in order for all these different layers to work together in a composable fashion, you require full openness from all the solution providers. For example, as business needs change, you may well want to plug in different analysis solutions. Equally, different low-code application frameworks - and SaaS applications outright — should be chosen for different business needs and business users.
Figure 2: Industrial customer requirements for high-impact asset optimization solutions are highly heterogeneous or require significant customization.
How should digitalization leaders work toward a more balanced stack — and thus avoid shiny proofs of concept that cannot be operationalized?
The digital twin only makes the contextualised data available to the user. To operationalise use cases — at what you refer to here as the third layer, that is focusing on the application development itself — what we really need is to have full confidence in the data presented to the application users. Basically, we’re talking about trust here. End-user trust in the data is absolutely needed, already at the most basic level of digital twin value.
Figure 3: End-user trust in the data is absolutely needed, already at the most basic level of digital twin value.
Trust — or confidence — in data accuracy is, of course, even more critical as we advance into predictions and prescriptions, and ultimately all the way to closed-loop decision execution. The latter is still many years out into the future. Right now, the foundational part we all need to focus on is trust in the data itself.
Trustworthy data delivery, in a real-time environment, to many different data consumers - this is what DataOps is all about. This may sound scripted now, but to operationalise digital twins in the industry, you really need trusted data delivery, focused on continuous data integration across all OT/IT/ET and visual data sources. This being the very definition of Industrial DataOps by Cognite.
Focusing on the class of industrial applications that is most commonly associated with digital twins in tabloid media — those addressing asset integrity, reliability, and maintenance with a rotating 3D model as the centrepiece — what can we expect to see here in terms of hard CFO value beyond impressive looking demo apps?
To be clear, what you are referring to here as ‘tabloid media digital twins with a 3D centrepiece’, already deliver very real value to many asset operators.
At the same time, and what I believe you’re pointing to with your question, the 3D CAD, lidar, or photogrammetry-based 3D reconstruction is certainly stealing a lot of the thunder from a much larger class of integrated industrial data use cases — or digital twin applications — without such data virtualization front end. Examples here include supply chain digital twins, well delivery digital twins, production optimization digital twins, and many more. All these with much more modest looking end-user application front ends.
For the high-value purposes of data science model development, the value of interconnected industrial data made possible by the data integration layer of the digital twin, does not depend on any 3D visual data representation. It is all about having an interconnected data model that can be queried programmatically. So yes, the highly visible tip of the iceberg on digital twin value is commanding an outsized share of digital twin attention.
Figure 4: Cognite Remote is a highly configurable business application that offers a dynamic operational digital twin to both locate, navigate, and understand data in real-world context, as well as to execute remote inspection operations.
In somewhat stark contrast to tabloid media — within the software developer community that is — the digital twin is increasingly understood as being the data model. Or more precisely speaking the graph data modelling service. Why is this?
Software developers — and data scientists by extension alike — all depend on data models to make analytical software applications work and scale.
Take, for example, someone developing a production optimization application for a fleet of gas fields. What they need is a strong domain API, that provides them instant access to the well data model, which contains all the relevant data for any given well. Regardless of where that data originates from. Or even where it is stored now, for performant querying in the cloud. And they need it all in domain language, not in the language of databases. So in our particular, simplified example, what powers this domain API is the data model for a well.
To provide this level of convenience to the developer and data scientist, the output of the work taking place at the data management and data integration layer of a digital twin architecture, is a data model, or rather, a set of data models, that remove the data complexity. This allows developers and data scientists to focus on application logic and algorithm development itself. And not on finding, transforming, integrating, and cleaning data before they can use it.
In short, the backbone of all digital twins is a unified data model that is developer-friendly. Graph of course lends itself well to such advanced data modelling.
Figure 5: Example of Cognite Data Fusion® (CDF) industrial graph used to model drilling & wells digital twin data model
What can we look forward to in terms of new value as we witness the visualization layer — the tabloid digital twin — become powered by the software developer community’s digital twin?
It already is - and when it comes to digital twin suppliers, this is where we’re going to see the wheat separating from the chaff. Those who really have their foundational data integration and data modelling in place will massively outperform those who have taken shortcuts on this most difficult, yet equally most critical part, of any future-proof digital twin platform architecture.
Trustworthy industrial data delivery, all the way up to the domain APIs that are powered by unified data models, is absolutely essential to a true digital twin delivery.
Let’s do a final lightning round question. Yes or no answers please: Will the term digital twin be around in 2025, or will it just be a series of apps powered by one or more data and analytics platforms?
Yes, the term digital twin will still be around.